A review on food recognition technology for health applications
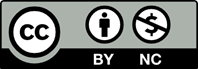
Food understanding from digital media has become a chal lenge with important applications in many different domains. On the other hand, food is a crucial part of human life since the health is strictly affected by diet. The impact of food in people life led Computer Vision specialists to develop new methods for automat ic food intake monitoring and food logging. In this review paper we provide an overview about automatic food intake monitoring, by focusing on technical aspects and Computer Vision works which solve the main involved tasks (i.e., classification, recogni tions, segmentation, etc.). Specifically, we conducted a systematic review on main scientific databases, including interdisciplinary databases (i.e., Scopus) as well as academic databases in the field of computer science that focus on topics related to image under standing (i.e., recognition, analysis, retrieval). The search queries were based on the following key words: “food recognition”, “food classification”, “food portion estimation”, “food logging” and “food image dataset”. A total of 434 papers have been retrieved. We excluded 329 works in the first screening and performed a new check for the remaining 105 papers. Then, we manually added 5 recent relevant studies. Our final selection includes 23 papers that present systems for automatic food intake monitoring, as well as 46 papers which addressed Computer Vision tasks related food images analysis which we consider essential for a comprehensive overview about this research topic. A discussion that highlights the limitations of this research field is reported in conclusions.
[1] Abdel-Hakim, A.E., & Farag, A.A. (2006). CSIFT: A SIFT descriptor with color invariant characteristics. Computer Vision and Pattern Recognition, 2, 1978–1983.
[2] Agrawal, M., Konolige, K., & Blas, M.R. (2008). CenSurE: Center Surround Extremas for Realtime Feature Detection and Matching. Lecture Notes in Computer Science, 5305, 102–115.
[3] Ahonen, T., Hadid, A., & Pietikäinen, M. (2006). Face description with local binary patterns: application to face recognition. Transactions on Pattern Analysis and Machine Intelligence, 28, 2037–2041.
[4] Ahuja, J., Montville, J.B., Omolewa-Tomobi, G., Heendeniya, K.Y., Martin, C.L., Steinfeldt, L.C., Anand, J., Adler, M.E., LaComb, R.P., & Moshfegh, A.J. (2012). USDA food and nutrient database for dietary studies, 5.0–documentation and user guide. US Department of Agriculture, Agricultural Research Service, Food Surveys Research Group: Beltsville, MD, USA.
[5] Aizawa, K., Silva, G.C., Ogawa, M., & Sato, Y. (2010). Food Log by snapping and processing images. 2010 16th International Conference on Virtual Systems and Multimedia, 71-74.
[6] Akpro Hippocrate, E. A., Suwa, H., Arakawa, Y., & Yasumoto, K. (2016). Food weight estimation using smartphone and cutlery. In: Proceedings of the First Workshop on IoT-enabled Healthcare and Wellness Technologies and Systems (IoT of Health ‘16). Association for Computing Machinery, New York, NY, USA, 9–14.
[7] Allegra, D., Anthimopoulos, M., Dehais, J., Lu, Y., Stanco, F., Farinella, G.M., & Mougiakakou, S. (2017). A multimedia database for automatic meal assessment systems. Lecture Notes in Computer Science, 10590, 471-478.
[8] Allegra, D., Erba, D., Farinella, G.M., Grazioso, G., Maci, P.D., Stanco, F., & Tomaselli, V. (2019). Learning to rank food images. Lecture Notes in Computer Science, 11752, pp. 629–639.
[9] Battiato, S., Farinella, G.M., Gallo, G., & Ravì, D. (2010). Exploiting textons distributions on spatial hierarchy for scene classification. Journal on Image and Video Processing, 2010, 919367.
[10] Battiato, S., Farinella, G.M., Puglisi, G., & Ravì, D. (2014). Aligning codebooks for near duplicate image detection. Multimedia Tools and Applications, 72, 1483–1506.
[11] Bay, H., Tuytelaars, T., & Van Gool, L. (2006). SURF: Speeded Up Robust Features. Lecture Notes in Computer Science, 3951, 404–417.
[12] Belongie, S., Alik, J., & Puzicha, J. (2002). Shape matching and object recognition using shape contexts. Transactions on Pattern Analysis and Machine Intelligence, 24, 509–522.
[13] Bettadapura, V., Thomaz, E., Parnami, A., Abowd, G.D., & Essa, I. (2015). leveraging context to support automated food recognition in restaurants. IEEE Winter Conference on Applications of Computer Vision, 580–587.
[14] Bosch, M., Schap, T., Zhu, F., Khanna, N., Boushey, C.J., Delp, E.J. (2011). Integrated database system for mobile dietary assessment and analysis. International Conference on Multimedia and Expo, 1–6.
[15] Bossard, L., Guillaumin, M., & Van Gool, L. (2014). Food-101 – Mining discriminative components with random forests. Lecture Notes in Computer Science, 8694, 446–461.
[16] Brosnan, T., & Sun, D.W. (2004). Improving quality inspection of food products by computer vision - A review. Journal of Food Engineering, 61, 3–16.
[17] Buemi, F., Massa, M., & Sandini, G. (1995). Agrobot: a robotic system for greenhouse operations. Workshop on Robotics in Agriculture and the Food Industry, pp. 172–184.
[18] Burghouts, G.J., & Geusebroek, J.M. (2009). Performance evaluation of local colour invariants. Computer Vision and Image Understanding, 113, 48–62.
[19] Cardenas-Weber, M., Hetzroni, A., & Miles, G.E. (1991). Machine vision to locate melons and guide robotic harvesting. American Society of Agricultural Engineers, p. 21.
[20] Chen, M., Dhingra, K., Wu, W., Yang, L., Sukthankar, R., & Yang, J. (2009). PFID: Pittsburgh fast-food image dataset. International Conference on Image Processing, pp. 289–292.
[21] Chen, M.Y., Yang, Y.H., Ho, C.J., Wang, S.H., Liu, S.M., Chang, E., Yeh, C.H., & Ouhyoung, M. (2012). Automatic Chinese food identification and quantity estimation. SIGGRAPH Asia 2012 Technical Briefs, 1–4.
[22] Chen, N., Lee, Y. Y., Rabb, M., & Schatz, B. (2010). Toward dietary assessment via mobile phone video cameras. AMIA . Annual Symposium proceedings. pp. 106–110.
[23] Dalal, N., & Triggs, B. Histograms of oriented gradients for human detection. 2005 IEEE Computer Society Conference on Computer Vision and Pattern Recognition (CVPR’05), San Diego, CA, USA, 1, pp. 886-893.
[24] Dehais, J., Shevchik, S., Diem, P., & Mougiakakou, S.G. (2013). Food volume computation for self dietary assessment applications. International Conference on Bioinformatics and Bioengineering.
[25] Delwiche, J.F. (2012). You eat with your eyes first. Physiology & Behavior, 107, 502–504.
[26] Deng, Y., & Manjunath, B.S. (2001). Unsupervised segmentation of color-texture regions in images and video. Transactions on Pattern Analysis and Machine Intelligence, 23, 800–810.
[27] Donadello, I., Dragoni, M. (2019). Ontology-driven food category classification in images. International Conference on Image Analysis and Processing. pp. 607–617.
[28] Du, C.J., & Sun, D.W. (2006). Learning techniques used in computer vision for food quality evaluation: a review. Journal of Food Engineering, 72, 39–55.
[29] Du, C.J.; & Sun, D.W. (2008). Multi-classification of pizza using computer vision and support vector machine. Journal of Food Engineering, 86, 232–242.
[30] Fang, S., Liu, C., Zhu, F., Delp, E.J., & Boushey, C.J. (2015). Single-view food portion estimation based on geometric models. 2015 IEEE International Symposium on Multimedia (ISM). pp. 385–390.
[31] Farinella, G.M., Allegra, D., Moltisanti, M., Stanco, F., & Battiato, S. (2016). Retrieval and classification of food images. Computers in Biology and Medicine, 77, 23–39.
[32] Farinella, G.M., Allegra, D., & Stanco, F. (2015). A benchmark dataset to study the representation of food images. Lecture Notes in Computer Science, 8927, pp. 584–599.
[33] Farinella, G.M., Allegra, D., & Stanco, F. (2015). On the exploitation of one class classification to distinguish food vs non-food images. Lecture Notes in Computer Science, 9281, 375–383.
[34] Farinella, G.M., Moltisanti, M., & Battiato, S. (2014). classifying food images represented as bag of textons. International Conference on Image Processing. pp. 5212–5216.
[35] Farinella, G.M., Moltisanti, M., & Battiato, S. (2015). Food recognition using consensus vocabularies. Lecture Notes in Computer Science, 9281, 384–392. doi:10.1007/978-3-31923222-5_47.
[36] Felzenszwalb, P.F., Girshick, R.B., Mcallester, D., & Ramanan, D. (2010). Object detection with discriminatively trained part-based models. Transactions on Pattern Analysis and Machine Intelligence, 32, 1627–1645.
[37] Fischler, M.A., & Bolles, R.C. (1981). Random sample consensus: a paradigm for model fitting with applications to image analysis and automated cartography. Communications of the ACM, 24, 381–395.
[38] Fontanellaz, M., Christodoulidis, S., & Mougiakakou, S. (2019). Self-attention and ingredient-attention based model for recipe retrieval from image queries. International Workshop on Multimedia Assisted Dietary Management. pp. 25–31.
[39] Foroni, F., Pergola, G., Argiris, G., & Rumiati, R.I. (2013). The FoodCast research image database (FRIDa). Frontiers in Human Neuroscience, 7.
[40] Gunasekaran, S. (1996). Computer vision technology for food quality assurance. Trends in Food Science & Technology, 7, 245–256.
[41] Hammond, R.A., Levine, R. (2010). The economic impact of obesity in the United States. Diabetes, Metabolic Syndrome and Obesity: Targets and Therapy, 3, 285–295.
[42] Herranz, L., Ruihan, X., & Shuqiang, J. (2015). A probabilistic model for food image recognition in restaurants. International Conference on Multimedia and Expo. pp. 1–6.
[43] Ho, T.K. (1995). Random decision forests. International Conference on Document Analysis and Recognition, 1, pp. 278–282.
[44] Hoashi, H., Joutou, T., & Yanai, K. (2010). Image recognition of 85 food categories by feature fusion. International Symposium on Multimedia, pp. 296–301.
[45] Hu, Y., Cheng, X., Chia, L.T., Xie, X., Rajan, D., & Tan, A.H. (2009). Coherent phrase model for efficient image near-duplicate retrieval. Transactions on Multimedia, 11, 1434–1445.
[46] Kawano, Y., & Yanai, K. (2014). Automatic expansion of a food image dataset leveraging existing categories with domain adaptation. Lecture Notes in Computer Science, 8927, pp. 3–17.
[47] Kawano, Y., & Yanai, K. (2014). Food image recognition with deep convolutional features. International Joint Conference on Pervasive and Ubiquitous Computing. pp. 589–593.
[48] Kawano, Y., & Yanai, K. (2013). Real-time mobile food recognition system. 2013 IEEE Conference on Computer Vision and Pattern Recognition Workshops. pp. 1–7.
[49] Kiliç, K., Boyaci, I.H., Köksel, H., & Küsmenoglu, I. (2007). A classification system for beans using computer vision system and artificial neural networks. Journal of Food Engineering, 78, 897–904.
[50] Killgore, W.D., & Yurgelun-Todd, D.A. (2005). Body mass predicts orbitofrontal activity during visual presentations of high-calorie foods. Neuroreport, 16, 859–863.
[51] Kitamura, K., De Silva, C., Yamasaki, T., & Aizawa, K. (2010). Image processing based approach to food balance analysis for personal food logging. International Conference on Multimedia and Expo. pp. 625–630.
[52] Kitamura, K., Yamasaki, T., & Aizawa, K. (2008). Food log by analyzing food images. International Conference on Multimedia. pp. 999–1000.
[53] Kitamura, K., Yamasaki, T., & Aizawa, K. (2009). FoodLog: capture, analysis and retrieval of personal food images via web. Workshop on Multimedia for cooking and eating activities. pp. 23–30.
[54] Kitamura, K., Yamasaki, T., & Aizawa, K. (2010). Personalization of food image analysis. International Conference on Virtual Systems and Multimedia. pp. 75–78.
[55] Kohonen, T. (1998). The self-organizing map. Neurocomputing, 21, 1–6.
[56] Kong, F., & Tan, J. (2012). DietCam: Automatic dietary assessment with mobile camera phones. Pervasive and Mobile Computing, 8, 147–163.
[57] Krizhevsky, A., Sutskever, I., & Hinton, G.E. (2012). ImageNet classification with deep convolutional neural networks. Neural Information Processing Systems. pp. 1097–1105.
[58] Lazebnik, S., Schmid, C., & Ponce, J. (2005). A sparse texture representation using local affine regions. Transactions on Pattern Analysis and Machine Intelligence, 27, 1265–1278.
[59] Lazebnik, S., Schmid, C., & Ponce, J. (2006). Beyond bags of features: spatial pyramid matching for recognizing natural scene categories. Conference onComputer Vision and Pattern Recognition, 2, pp. 2169–2178.
[60] Levi, P., Falla, A., & Pappalardo, R. (1988). Image controlled robotics applied to citrus fruit harvesting. International Conference on Robot Vision and Sensory Controls. pp. 2–4.
[61] Liu, J., Johns, E., Atallah, L., Pettitt, C., Lo, B., Frost, G., & Yang, G.Z. (2012). An intelligent food-intake monitoring system using wearable sensors. International Conference on Wearable and Implantable Body Sensor Networks.
[62] Lowe, D.G. (2004). Distinctive image features from scale-invariant keypoints. International Journal of Computer Vision, 60, 91–110.
[63] Lu, Y., Allegra, D., Anthimopoulos, M., Stanco, F., Farinella, G.M., & Mougiakakou, S. (2018). A Multi-task learning approach for meal assessment. Joint Workshop on Multimedia for Cooking and Eating Activities and Multimedia Assisted Dietary Management. pp. 46–52.
[64] Lu, Y., Stathopoulou, T., Vasiloglou, M.F., Christodoulidis, S., Blum, B., Walser, T., Meier, V., Stanga, Z., & Mougiakakou, S. (2019). an artificial intelligence-based system for nutrient intake assessment of hospitalised patients. International Conference of the IEEE Engineering in Medicine and Biology Society. pp. 5696–5699.
[65] Marĉelja, S. (1980). Mathematical description of the responses of simple cortical cells. Journal of the Optical Society of America, 70, 1297–1300.
[66] Marin, J., Biswas, A., Ofli, F., Hynes, N., Salvador, A., Aytar, Y., Weber, I., & Torralba, A. (2019). Recipe1M+: A dataset for learning cross-modal embeddings for cooking recipes and food Images. IEEE transactions on pattern analysis and machine intelligence.
[67] Maruyama, Y., De Silva, G.C., Yamasaki, T., & Aizawa, K. (2010). Personalization of food image analysis. International Conference on Virtual Systems and Multimedia. pp. 75–78.
[68] Matas, J., Chum, O., Urban, M., & Pajdla, T. (2004). Robust wide-baseline stereo from maximally stable extremal regions. Image and Vision Computing, 22, 761–767.
[69] Medic, N., Ziauddeen, H., Forwood, S.E., Davies, K.M., Ahern, A.L., Jebb, S.A., Marteau, T.M., & Fletcher, P.C. (2016). The presence of real food usurps hypothetical health value judgment in overweight people. eNeuro, 3, 0025–16.
[70] Meyers, A., Johnston, N., Rathod, V., Korattikara, A., Gorban, A., Silberman, N., Guadarrama, S., Papandreou, G., Huang, J., & Murphy, K.P. (2015). Im2Calories: towards an automated mobile vision food diary. Available from: https://static.googleusercontent.com/media/research.google.co m/it//pubs/archive/44321.pdf.
[71] Min, W., Jiang, S., Liu, L., Rui, Y., & Jain, R. (2019). A survey on food computing. ACM Computing Surveys (CSUR), 52, 92.
[72] Munkevik, P., Duckett, T., & Hall, G. (2007). A computer vision system for appearance-based descriptive sensory evaluation of meals. Journal of Food Engineering, 78, 246–256.
[73] Munkevik, P., Duckett, T., & Hall, G. (2004). Vision system learning for ready meal characterisation. International Conference on Engineering and Food.
[74] Nguyen, D.T., Zong, Z., Ogunbona, P., & Li, W. (2010). Object detection using non-redundant local binary patterns. International Conference on Image Processing. pp. 4609–4612.
[75] Nishida, C., Uauy, R., Kumanyika, S., & Shetty, P. (2004). The joint WHO/FAO expert consultation on diet, nutrition and the prevention of chronic diseases: process, product and policy implications. Public health nutrition, 7(1a), 245-250.
[76] Nistér, D., & Stewénius, H. Scalable recognition with a vocabulary tree. (2006). Computer Vision and Pattern Recognition, 2, 2161–2168.
[77] Ortis, A., Farinella, G. M., & Battiato, S. (2020). Survey on visual sentiment analysis. IET Image Processing, 14(8), 1440-1456.
[78] Ortis, A., Farinella, G.M., & Battiato, S. (2019). Predicting social image popularity dynamics at time zero. IEEE Access, 1–1.
[79] Ortis, A., Farinella, G.M., Torrisi, G., & Battiato, S. (2020). Exploiting objective text description of images for visual sentiment analysis. Multimedia Tools and Applications.
[80] Parrish, E.A., & Goksel, K.A. (1977). Pictorial pattern recognition applied to fruit harvesting. Transactions of the American Society of Agricultural and Biological Engineers, 20, 822–827.
[81] Perronnin, F., & Dance, C. (2007). Fisher Kernels on visual vocabularies for image categorization. Computer Vision and Pattern Recognition, pp. 1–8.
[82] Perronnin, F., Sánchez, J., & Mensink, T. (2010). Improving the Fisher Kernel for large-scale image classification. Lecture Notes in Computer Science, 6314, pp. 143–156.
[83] Petit, O., Cheok, A.D., Oullier, O. (2016). Can food porn make us slim? how brains of consumers react to food in digital environments. Integrative Food, Nutrition and Metabolism, 3, 251–255.
[84] Pham, C., Jackson, D., Schöning, J., Bartindale, T., Plotz, T., & Olivier, P. (2013). FoodBoard: surface contact imaging for food recognition. International Joint Conference on Pervasive and Ubiquitous Computing. pp. 749–752.
[85] Plebe, A., & Grasso, G. (2019). The unbearable shallow understanding of deep learning. Minds and Machines, 29(4), 515-553.
[86] Pouladzadeh, P., Yassine, A., & Shirmohammadi, S. (2015). FooDD: Food Detection Dataset for Calorie Measurement Using Food Images. Lecture Notes in Computer Science, 9281, 441–448.
[87] Puri, M., Zhu, Z., Yu, Q., Divakaran, A., & Sawhney, H. (2009). Recognition and volume estimation of food intake using a mobile device. Workshop on Applications of Computer Vision.
[88] Qi, X., Xiao, R., Li, C.G., Qiao, Y., Guo, J., & Tang, X. (2014). Pairwise rotation invariant co-occurrence local binary pattern. Transactions on Pattern Analysis and Machine Intelligence, 36, 2199–2213.
[89] Ragusa, F., Tomaselli, V., Furnari, A., Battiato, S., & Farinella, G.M. (2016). Food vs non-food classification. International Workshop on Multimedia Assisted Dietary Management. pp. 77–81.
[90] Rahmana, M.H., Pickering, M.R., Kerr, D., Boushey, C.J., & Delp, E.J. (2012). a new texture feature for improved food recognition accuracy in a mobile phone based dietary assessment system. International Conference on Multimedia and Expo Workshops. pp. 418–423.
[91] Ravì, D., Lo, B., & Yang, G.Z. (2015). Real-time food intake classification and energy expenditure estimation on a mobile device. International Conference on Wearable and Implantable Body Sensor Networks.
[92] Rich, A.J. (1981). A programmable calculator system for the estimation of nutritional intake of hospital patients. The American Journal of Clinical Nutrition, 34, 2276–2279.
[93] Rosenbaum, M., Sy, M., Pavlovich, K., Leibel, R.L., & Hirsch, J. (2008). Leptin reverses weight loss–induced changes in regional neural activity responses to visual food stimuli. The Journal of Clinical Investigation, 118, 2583–2591.
[94] Ruihan, X., Herranz, L., Shuqiang, J., Shuang, W., Xinhang, S., & Jain, R. (2015). Geolocalized modeling for dish recognition. Transactions on Multimedia, 17, 1187–1199.
[95] Sánchez, J., Perronnin, F., Mensink, T., & Verbeek, J. (2013). Image classification with the fisher vector: theory and practice. International Journal of Computer Vision, 105, 222–245.
[96] Shotton, J., Johnson, M., Cipolla, R. (2013). Semantic texton forests for image categorization and segmentation. Conference in Advances in Computer Vision and Pattern Recognition. pp. 211–227.
[97] Shroff, G., Smailagic, A., & Siewiorek, D. P. (2008). Wearable context-aware food recognition for calorie monitoring. 12th IEEE International Symposium on Wearable Computers. pp. 119-120. IEEE.
[98] Slaughter, D.C, & Harrell, R.C. (1989). Discriminating fruit for robotic harvest using color in natural outdoor scenes. Transactions of the American Society of Agricultural and Biological Engineers, 32, 757–763.
[99] Sun, D.W. (2000). Inspecting pizza topping percentage and distribution by a computer vision method. Journal of Food Engineering, 44, 245–249.
[100] Sun, M., Burke, L.E., Mao, Z.H., Chen, Y., Chen, H.C., Bai, Y., Li, Y., Li, C., & Jia, W. (2014). eButton: a wearable computer for health monitoring and personal assistance. Proceedings of the 51st Annual Design Automation Conference. ACM. pp. 1–6.
[101] Suthumchai, N., Thongsukh, S., Yusuksataporn, P., & Tangsripairoj, S. (2016). FoodForCare: An Android application for self-care with healthy food. International Student Project Conference (ICT-ISPC). pp. 89–92.
[102] Tola, E., Lepetit, V., & Fua, P. (2009). DAISY: An efficient dense descriptor applied to wide-baseline stereo. Transactions on Pattern Analysis and Machine Intelligence, 32, 815–830.
[103] Topchy, A., Jain, A.K., & Punch, W. (2005). Clustering ensembles: models of consensus and weak partitions. Transactions on Pattern Analysis and Machine Intelligence, 27, 1866–1881.
[104] Varma, M., & Ray, D. (2007). Learning The Discriminative Power-Invariance Trade-Off. International Conference on Computer Vision. pp. 1–8.
[105] Varma, M., & Zisserman, A. (2005). A Statistical Approach to Texture Classification from Single Images. International Journal of Computer Vision, 62, 61–81.
[106] Vedaldi, A., & Zisserman, A. (2012). Efficient additive kernels via explicit feature maps. Transactions on Pattern Analysis and Machine Intelligence, 34, 480–492.
[107] Wenz, A., Jäckle, A., & Couper, M.P. (2019). Willingness to use mobile technologies for data collection in a probability household panel. Survey Research Methods, 13, 1–22.
[108] Wright, P.D., Shearing, G., Rich, A.J., & Johnston, I. (1978) The role of a computer in
[108] Wright, P.D., Shearing, G., Rich, A.J., & Johnston, I. (1978) The role of a computer in the management of clinical parenteral nutrition. Journal of Parenteral and Enteral Nutrition, 2, 652–657.
[109] Wu, W., & Yang, J. (2009). Food recognition using statistics of pairwise local features. International Conference on Multimedia and Expo. pp. 1210–1213.
[110] Xin, W., Kumar, D., Thome, N., Cord, M., & Precioso, F. (2015). Recipe recognition with large multimodal food dataset. International Conference on Multimedia Expo Workshops. pp. 1–6.
[111] Xu, K., Zhou, R., Takei, K., & Hong, M. (2019). Toward Flexible Surface-Enhanced Raman Scattering (SERS) Sensors for Point-of-Care Diagnostics. Advanced Science, 6.
[112] Xu, M.L., Gao, Y., Han, X.X. & Zhao, B. (2017). detection of pesticide residues in food using surface-enhanced raman spectrometry: a review. Journal of Agricultural and Food Chemistry, 65, 6719–6726.
[113] Yanai, K., & Joutou, T. (2009). SURF: Speeded Up Robust Features. International Conference on Image Processing. pp. 285–288.
[114] Yanai, K., & Kawano, Y. (2015). Food image recognition using deep convolutional network with pre-training and fine-tuning. International Conference on Multimedia & Expo Workshops. pp. 1–6.
[115] Yang, S., Chen, M., Pomerleau, D., & Sukthankar, R. (2010). Food recognition using statistics of pairwise local features. Conference on Computer Vision and Pattern Recognition. pp. 2249–2256.
[116] Zhang, W., Yu, Q., Siddiquie, B., Divakaran, A., & Sawhney, H. (2015). “Snap-n-Eat” food recognition and nutrition estimation on a smartphone. Journal of diabetes science and technology, 9, 525–533.
[117] Zhu, F., Bosch, M., Woo, I., Kim, S., Boushey, C.J., Ebert, D.S., & Delp, E.J. (2010). The use of mobile devices in aiding dietary assessment and evaluation. European Journal of Clinical Nutrition, 4, 756–766.
[118] Zong, Z., Nguyen, D.T., Ogunbona, P., & Li, W. (2010). On the combination of local texture and global structure for food classification. International Symposium on Multimedia. pp. 204–211.